Jump to a key chapter
Perfect Bayesian Equilibrium - Definition
In the study of microeconomics and game theory, the concept of Perfect Bayesian Equilibrium (PBE) plays a significant role. It helps in understanding strategies where players have incomplete information. PBE combines elements of Nash Equilibrium with Bayesian inference to model how players form beliefs and strategies based on observed actions.
A Perfect Bayesian Equilibrium is a strategy profile and belief specification that satisfies two conditions:
- Players must be sequentially rational. This means each player's strategy maximizes their expected payoff, given their beliefs and other players' strategies.
- Players' beliefs must be updated using Bayes' Rule, whenever possible, relative to the strategy profile.
Understanding PBE requires a basic understanding of probability, actions, and payoffs. In PBE, each player considers their own strategy as well as the strategy of others. Each player seeks to maximize their expected payoff, even with incomplete information. The equilibrium is 'perfect' because players adjust their strategies based on observed actions and updated beliefs.
Consider a game where you and another player are bidders in an auction. You're both trying to guess the value of an item up for bid without knowing the true value nor each other's valuation. A Perfect Bayesian Equilibrium in this auction would involve strategies where both you and the other player optimize bids based on past bidding rounds and conditional probability, leading to logically updated beliefs about the item's value.
Remember, PBE involves the logical updating of beliefs using Bayes' Rule. This makes it more realistic compared to traditional Nash Equilibriums in games with incomplete information.
Let's delve deeper into how beliefs are modeled in a Perfect Bayesian Equilibrium. Each player's beliefs are best represented as a probability distribution over types. In a game with nature, a player's type could represent private information like costs or negotiated prices. The updating of these beliefs uses Bayes' Rule, stated as: \[ P(\text{Type | Information}) = \frac{P(\text{Information | Type}) \cdot P(\text{Type})}{P(\text{Information})} \] This rule informs how players adjust their perceptions about potential types, given the actions and observed outcomes. By continuously revising these beliefs, players can adapt and tailor strategies even in the varied landscape of a dynamic game model. This adaptability is what lends PBE its 'perfect' status in game theoretic analysis.
Perfect Bayesian Equilibrium Example
Analyzing an example of Perfect Bayesian Equilibrium (PBE) can provide clarity on how it operates in game theory. In games where information is incomplete, players rely on observed actions and Bayesian updating to form optimal strategies. Let's explore a practical scenario.
Imagine a signaling game involving a job market. In this game, there is an employer and a potential employee. The employee has two types: high ability (H) and low ability (L), known only to themselves. The employer observes the education level attained by the employee but not their true ability.Here's a potential equilibrium strategy:
- The high ability employee chooses a high education level since the cost of education is relatively low for them.
- The low ability employee chooses a low education level due to the high cost of education for them.
- The employer, upon observing a high education level, offers a high wage, believing the candidate is of high ability with updated beliefs.
In this signaling game, let's delve into the mathematics behind the updated beliefs using Bayes' Rule.Suppose the probability that an employee is of high ability given they have a high education level is represented as: \[ P(H | \text{High Education}) = \frac{P(\text{High Education} | H) \cdot P(H)}{P(\text{High Education})} \] Here:
- P(H | High Education) is the probability the candidate is high ability, given they chose high education.
- P(High Education | H) is the likelihood of choosing high education given high ability.
- P(H) is the prior probability of a candidate having high ability.
- P(High Education) is the probability of high education being chosen overall.
In signaling games like this, education acts as a costly signal that varies in cost depending on ability. PBE relies on the assumption that these signals are verifiable and honest.
Perfect Bayesian Equilibrium Problem Set
Embarking on problem sets involving Perfect Bayesian Equilibrium (PBE) allows you to apply theoretical concepts practically. These problems are designed to test your understanding of game theoretic models, focusing on how players form beliefs and strategies based on observed actions in games with incomplete information.
Perfect Bayesian Equilibrium Problem 1
In Problem 1, consider a basic entry game scenario between an incumbent firm and a potential entrant. The entrant can either choose to enter a market or stay out. The incumbent firm can either accommodate the entrant or fight to deter entry. However, the entrant is not sure whether the firm can fight (Strong type) or can only accommodate (Weak type).
Entrant | Stay Out | Enter |
Incumbent (Strong) | (0, 0) | (-1, 1) |
Incumbent (Weak) | (0, 0) | (0, 1) |
A Perfect Bayesian Equilibrium in this scenario involves:
- Strategies for both the incumbent and the entrant that are sequentially rational
- Updating beliefs by the entrant about the type of incumbent using observed actions and Bayes' Rule
Suppose the incumbent decides to fight only if it is strong. Given the entrant's belief and choice to enter, he observes a fight. He updates his belief using Bayes' Rule: \[ P(\text{Strong} | \text{Fight}) = \frac{P(\text{Fight} | \text{Strong}) \times P(\text{Strong})}{P(\text{Fight})} \] where:
- \text{Fight} | \text{Strong} = 1, meaning the strong always fights.
- P(\text{Strong}) = 0.6
- P(\text{Fight)} is the probability of observing a fight.
A deeper dive into strategic manipulation in PBE reveals that incumbents might want to bluff to establish a reputation. For instance, a weak incumbent might fight initially to mimic the behavior of a strong incumbent, tricking the entrant into believing they are indeed strong. Over time, these shifts in beliefs can profoundly impact market dynamics and the outcome of such strategic games.Mathematically, if a weak incumbent fights with some probability \( q \), the entrant's posterior beliefs evolve in a more complex manner: \[ P(\text{Strong} | \text{Fight}) = \frac{1 \times P(\text{Strong})}{(1 \times P(\text{Strong}) + q \times P(\text{Weak}))} \] This equilibrium reflects both the idea of pooling and separating strategies.
In entry games, it is crucial to understand the roles of reputation and bluffing, as these can drive players' actions in a strategic game setting.
Perfect Bayesian Equilibrium Problem 2
In Problem 2, consider a trust game involving two players: a truster and a trustee. The truster decides to trust or not, and the trustee can either honor the trust or betray it. The trustee has private information about their type: trustworthy or untrustworthy.
Truster | Don't Trust | Trust |
Trustee (Trustworthy) | (0, 0) | (2, 1) |
Trustee (Untrustworthy) | (0, 0) | (-1, 3) |
A Perfect Bayesian Equilibrium in this context would require:
- Both players adopt a sequentially rational strategy
- The truster's belief updates using observed actions and Bayes' Rule, influencing their decision to trust or not
In a scenario where the truster decides to trust, and the trustee honors this trust, the truster updates their beliefs: \[ P(\text{Trustworthy} | \text{Honors}) = \frac{P(\text{Honors} | \text{Trustworthy}) \times P(\text{Trustworthy})}{P(\text{Honors})} \]This approach helps the truster decide the long-term strategy regarding repeated interactions with the trustee.
The trust game is particularly insightful when considering repeated games, where reputation plays a pivotal role. Over time, a trustee who honors trust builds a positive reputation, which can enhance future interactions. Mathematically, suppose the trustee honors trust with a probability \( p \) when trustworthy and attempts to mimic trustworthy behavior with probability \( r \) when untrustworthy: \[ P(\text{Trustworthy} | \text{Honors}) = \frac{p \times P(\text{Trustworthy})}{(p \times P(\text{Trustworthy}) + r \times P(\text{Untrustworthy}))} \] This Bayesian updating guides the truster towards optimal decisions in a dynamic setting.
Assessing the potential benefits of trust can be more rewarding than the perceived immediate gains of betrayal in repeated trust games.
Perfect Bayesian Equilibrium Signaling Game
In a Perfect Bayesian Equilibrium (PBE) signaling game, players with private information send signals to influence the beliefs and actions of others. They operate under incomplete information, making it essential to update beliefs based on observed signals. This makes signaling games a fascinating application of PBE.
Concept of Signaling in PBE
Signaling occurs when a player (sender) moves first, sending a signal to another player (receiver), who then takes action based on the signal and updated beliefs. The sender's type, known only to them, influences their choice of signal. Signals might involve costly actions that differentiate types, playing a vital role in strategic interactions in economics.
A signaling game involves:
- A sender with different types, unknown to the receiver
- A signal chosen by the sender, observed by the receiver
- A response or action by the receiver based on the signal
- Belief updates by the receiver based on observed signals and Bayes' Rule
Consider a job market scenario. An applicant (sender) can either have high skill (H) or low skill (L). They choose a level of education as a signal. An employer (receiver) observes the education level and decides on the wage offer.
Applicant Type | Signal: Education Level |
High Skill (H) | High Education |
Low Skill (L) | Low Education |
In this signaling game, education acts as a costly but credible signal, helping separate high and low skill types. The cost of acquiring an education level for low skill individuals is higher, discouraging them from mimicking high-skill signals.
Not all signals are equally credible. The effectiveness of a signal depends on its cost structure and the ability it has to differentiate between types.
Let's explore how belief updating using Bayes' Rule is mathematically expressed in signaling games with PBE. Suppose the employer perceives an education signal and updates their beliefs about the applicant's type:The probability that the applicant is high-skilled given they pursue high education is represented as: \[ P(H | \text{High Education}) = \frac{P(\text{High Education} | H) \times P(H)}{P(\text{High Education})} \]This equation highlights:
- P(H | High Education): The updated probability the applicant is high-skilled, given high education.
- P(High Education | H): The likelihood of a high-skilled individual choosing high education.
- P(H): The prior probability that any applicant is of high skill.
- P(High Education): The total probability someone gets high education.
Application of Perfect Bayesian Equilibrium
The Perfect Bayesian Equilibrium (PBE) is a critical tool in microeconomic theory and game theory, particularly in games where players have private information. PBE is widely applicable across various domains, including economics, political science, and biology. Its utility arises from its ability to model strategic interactions where beliefs and sequential moves play pivotal roles.
Economic Markets and Auctions
In economic markets, particularly in auctions, PBE is used to predict how bidders strategize based on available information. Consider a sealed-bid auction where each bidder has private valuations for an item. Bidders must form strategies based on their valuations and beliefs about rivals' valuations.Suppose bidders update beliefs about the value of the item using signals like other bids or public information. In a common value auction, beliefs about the item's worth are adjusted, influencing bid strategies and ultimately determining the auction's outcome.
In an auction game, each bidder applies PBE by:
- Assessing their private valuation \( V_i \)
- Observing others' bids and signals
- Updating beliefs with Bayes' Rule
- Formulating a bidding strategy that maximizes expected utility
By employing PBE, auction designers can predict outcomes and modify rules for efficiency or revenue-maximization. It accommodates both private information and strategic interactions inherent in auction formats.
Political Science Applications
In political science, PBE is employed to model scenarios such as electoral strategies and lobbying, where players have goals set amidst uncertainties about voter behaviors or policy impacts.For example, political candidates choose policies (signals) to appeal to a voter base with private preferences. They predict voter response by updating beliefs on voter types and preferences, shaping campaign strategies accordingly.
Consider an election where two parties A and B choose platforms based on beliefs about voter preferences. Both parties wish to maximize voter turnout and support:\[ P(\text{Platform | Victory}) = \frac{P(\text{Victory | Platform}) \times P(\text{Platform})}{P(\text{Victory})} \]The calculus involves:
- \( P(\text{Platform | Victory}) \): The probability of choosing a platform given victory
- \( P(\text{Victory | Platform}) \): The likelihood of winning with a platform
- \( P(\text{Platform}) \): Prior probability of selecting that platform
Biological Interactions
PBE extends to biological sciences, particularly in studying evolutionary biology and behavior. Animal signaling, where organisms communicate through behaviors or signals, often resembles game-theoretic scenarios.For instance, in predator-prey dynamics, predators may rely on signals sent by prey to assess fitness. Prey might evolve to send signals representing strength or toxicity, with predators adapting beliefs and strategies accordingly.
In evolutionary games, signals that seem costly or risky to produce (such as bright plumage in birds) can be reliable indicators of underlying fitness, impacting survival and reproductive success.
A biological signaling game may involve:
- Prey signaling strength through vibrant displays
- Predators applying Bayesian updates to assess prey type
- Formulating hunting strategies to optimize energy expenditure
Bayesian Nash Equilibrium vs Perfect Bayesian Equilibrium
In game theory, understanding the differences between Bayesian Nash Equilibrium (BNE) and Perfect Bayesian Equilibrium (PBE) is essential as both deal with games featuring incomplete information. While they share similarities, the distinction lies in their approach to players' beliefs and strategies. Let's delve into these concepts to grasp how they uniquely apply in economic analysis and strategic decision-making scenarios.
Bayesian Nash Equilibrium
The concept of Bayesian Nash Equilibrium extends the Nash Equilibrium to accommodate incomplete information. In BNE, players have beliefs about the types or strategies of other players, with each player choosing a strategy that maximizes their expected utility given these beliefs. The equilibrium occurs when no player can unilaterally deviate and achieve higher payoff, assuming their beliefs are correct.
- Each player's strategy maximizes expected utility based on private information and beliefs.
- Equilibrium is static, meaning strategies don't change after observing others' actions.
In a game with players indexed by \( i \in N \), a Bayesian Nash Equilibrium is a set of strategies \( (s_1^*, s_2^*, ..., s_n^*) \) such that: \[ s_i^* \text{ maximizes } E[U_i(s_i, s_{-i}) | \text{information}^i] \text{ for all } i \] where \( s_{-i} \) are the strategies of all players except \( i \), \( U_i \) is the utility function of player \( i \), and \( E \text{ denotes expectation} \).
Consider an auction where players bid for an item with private valuations. Each player forms beliefs about other bidders' valuations and bids accordingly. If all bidders' strategies maximize their expected payoff, the set of strategies forms a Bayesian Nash Equilibrium.
Bayesian Nash Equilibrium assumes common prior beliefs among players, ensuring everyone's expectations are aligned with shared information.
Perfect Bayesian Equilibrium
The Perfect Bayesian Equilibrium refines the concept of Bayesian Nash Equilibrium by incorporating sequential rationality and belief updating. PBE is relevant in dynamic games where players' decisions are observed, often prompting revisions in beliefs and subsequent strategies.
- PBE starts with prior beliefs which are updated through Bayes' Rule based on observed actions.
- Players' strategies are sequentially rational, meaning choices are optimal at each decision point.
In a signaling game, an employee chooses an education level (signal) to convey their skill to an employer. Based on the education signal, the employer updates beliefs and offers a wage. The employee's and employer's strategies form a PBE if they are rational and consistent with each other's beliefs.
In more complex setups, comparing BNE and PBE involves how players form expectations and adapt strategies.Let's consider a mathematical representation of belief updating in PBE using \text{Bayes' Rule}:For a belief that a player is of type \( \theta \) given an action \( a \), the updated belief is:\[ P(\theta | a) = \frac{P(a | \theta) \times P(\theta)}{P(a)} \]Here,
- \( P(\theta | a) \) is the posterior probability of type \( \theta \).
- \( P(a | \theta) \) is the likelihood of action \text{a} given type \ \( \theta. \)
- \( P(\theta) \) is the prior probability of type \( \theta \).
- \( P(a) \) is the total probability of action \text{a}.
PBE is especially useful in multi-stage games where players strategically adapt and respond to new information over time.
perfect Bayesian equilibrium - Key takeaways
- Perfect Bayesian Equilibrium (PBE) integrates Nash Equilibrium and Bayesian inference to address games with incomplete information, focusing on players' beliefs and strategies.
- A PBE requires sequentially rational strategies maximizing expected payoffs and belief updates using Bayes' Rule based on observed actions.
- Example of PBE: In an auction, bidders optimize their bids by updating beliefs about an item's value through previous bids and probabilities.
- PBE is applicable in various domains, including economic markets and evolutionary biology, enhancing strategic decision-making under uncertainty.
- Bayesian Nash Equilibrium vs Perfect Bayesian Equilibrium: The main difference is PBE's focus on sequential moves and belief updates, while BNE assumes static strategies with common beliefs.
- PBE signaling games involve sending signals under uncertainty to influence others' beliefs and actions, using costly signals to differentiate between types.
Learn faster with the 12 flashcards about perfect Bayesian equilibrium
Sign up for free to gain access to all our flashcards.
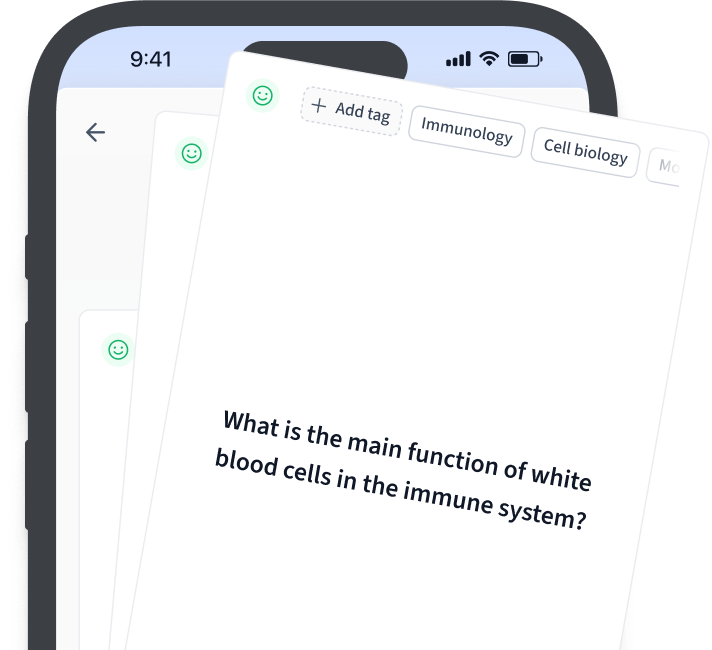
Frequently Asked Questions about perfect Bayesian equilibrium
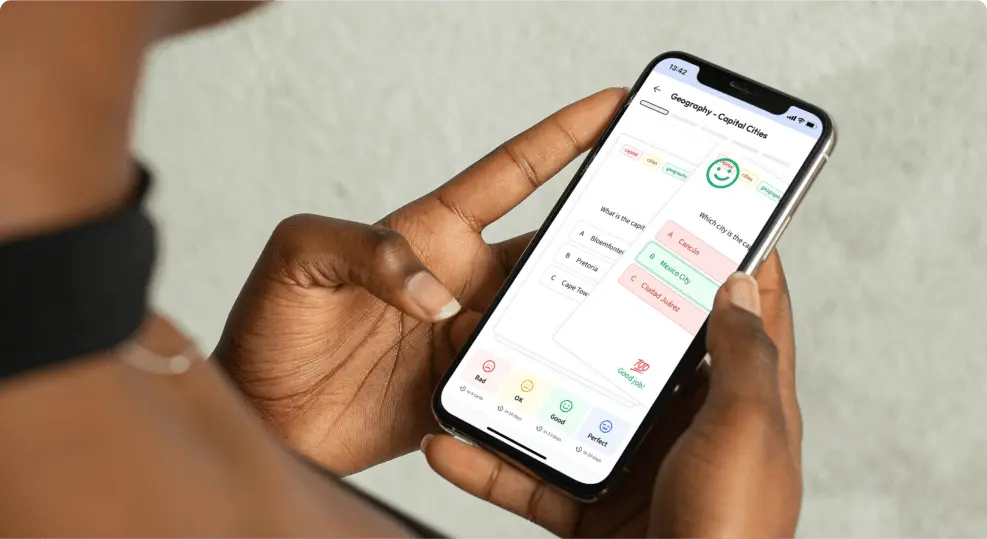
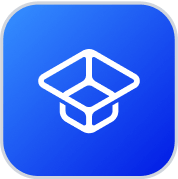
About StudySmarter
StudySmarter is a globally recognized educational technology company, offering a holistic learning platform designed for students of all ages and educational levels. Our platform provides learning support for a wide range of subjects, including STEM, Social Sciences, and Languages and also helps students to successfully master various tests and exams worldwide, such as GCSE, A Level, SAT, ACT, Abitur, and more. We offer an extensive library of learning materials, including interactive flashcards, comprehensive textbook solutions, and detailed explanations. The cutting-edge technology and tools we provide help students create their own learning materials. StudySmarter’s content is not only expert-verified but also regularly updated to ensure accuracy and relevance.
Learn more