Jump to a key chapter
Dynamic Games in Microeconomics Definition
Dynamic games in microeconomics involve strategic interactions where decisions are made over multiple periods. Unlike static games, these dynamic scenarios allow players to consider past actions when making current decisions, thereby introducing time and information into their decision-making processes.In a dynamic game, players often have opportunities to revise their strategies as the game progresses, leading to different outcomes based on how information is revealed and used. This makes dynamic games a critical component of microeconomic analysis.
Key Characteristics of Dynamic Games
Dynamic games are distinct due to several key characteristics:
- Stages: Unlike static games, dynamic games unfold in stages over time, each stage influencing the next.
- Strategy Revision: Players can continuously revise their strategies based on new information and past outcomes.
- Backward Induction: Used to determine optimal strategies, players start from the last stage and work backwards.
- Perfect vs. Imperfect Information: Games are categorized based on whether all past actions are known to all players.
Consider a simple two-stage game involving two firms competing in a market. In the first stage, Firm A decides whether to enter the market. If Firm A enters, Firm B chooses in the second stage whether to compete or collude. The potential outcomes can be analyzed using backward induction. Suppose Firm B has the following payoff matrix in the second stage:
Firm B Strategy | Compete | Collude |
---|---|---|
Firm A Enters | 1, 1 | 3, 2 |
Firm A Does Not Enter | 0, 0 | 0, 0 |
Importance of Time and Information in Dynamic Games
Time and information are of paramount importance in dynamic games because they determine how future actions and outcomes are shaped. In many real-world scenarios, decisions made today affect the alternatives available tomorrow. This requires a strategic analysis of not only the present situation but also possible future responses and adaptations.The concept of sequential rationality is vital. This involves making decisions that are optimal given the entire history of play. Information can be complete or incomplete, perfect or imperfect:
- Complete Information: When all players know the structure and payoffs of the game.
- Incomplete Information: Some elements, such as payoffs, are unknown to players.
- Perfect Information: Every past action is visible to all players.
- Imperfect Information: Not all actions are observed, leading to uncertainty.
Let's explore the Hanabi game, a cooperative card game where players hold their cards so that they can only be seen by others. This represents an environment of imperfect information — players have to infer the state of the game based on limited clues given by others. Hanabi's mechanics require strategic communication and use of information over time to achieve optimal outcomes. This aspect of dynamic games models real-world strategic challenges, where decision-makers must operate under conditions of uncertainty and imperfect knowledge. The challenge becomes aligning on common strategies with imperfect signals, which directly mirrors many market situations and negotiations in economic contexts.
Non Cooperative Dynamic Game Theory
In the realm of microeconomics, Non Cooperative Dynamic Game Theory focuses on strategic interactions where players make sequential decisions independently. These players aim to maximize their own payoff without forming binding agreements with others. This aspect of game theory profoundly influences economic behavior and policy design, as it models competitive environments where cooperation is either not feasible or not enforced. By analyzing the actions and reactions of individual firms or players over time, you can predict and understand complex economic phenomena.
Strategies in Non Cooperative Dynamic Games
Strategies in non cooperative dynamic games are critical as they guide players in making decisions that affect future outcomes. These strategies consider multiple stages of play, incorporating both the current state of the game and possible future scenarios. The most effective strategies often utilize principles like backward induction to anticipate the best responses at each stage and arrive at a subgame perfect equilibrium.The subgame perfect equilibrium is an extension of Nash equilibrium for dynamic games, ensuring that the player's strategy remains optimal even in every subsequence of the game. For example, consider the Stackelberg competition model, where a leader firm first moves and a follower firm responds. The leader anticipates the optimal reaction of the follower when choosing its initial output level.Here's a simplified model of Stackelberg competition:
- Leader chooses its production level, qL.
- Follower observes qL and chooses its production level, qF.
- Market price is determined by the total output, P(Q) = a - bQ, where Q = qL + qF.
A Subgame Perfect Equilibrium is a refinement of Nash Equilibrium applicable in dynamic games. It represents a strategy profile that yields an optimal decision for every subgame, ensuring that no player has an incentive to deviate from their chosen strategy at any stage.
In a classic job market example, two firms decide whether to invest in training for employees or hire externally. The game proceeds as follows:
- Stage 1: Firm A decides on investment.
- Stage 2: Firm B observes and decides similarly.
Consider the Centipede Game, a theoretical model illustrating conflict between immediate gain and potential long-term benefits. In this game, two players alternately decide whether to take a larger share of a growing pot or pass to let it increase further. The backward induction principle suggests terminating early, despite higher collective payoffs by continuing. Such games highlight how non cooperative strategies prioritize individual rationality over collective gain and are critical for understanding the logic of strategic decision-making in competing interests. Despite its simplicity, the Centipede Game illustrates the complexities faced in strategic environments, especially those characterized by distrust or competitive pressures.
Real-World Applications of Non Cooperative Dynamic Game Theory
Non cooperative dynamic game theory finds application across numerous real-world economic contexts. Understanding these games can provide insights into strategic behaviors in industries, international trade, and even political decisions. These games showcase how decisions affect competitive dynamics and market outcomes.In the telecommunications industry, firms make strategic decisions regarding pricing and capacity investing, considering the potential responses of their rivals. In international trade negotiations, countries formulate policies by anticipating the actions of other nations, often balancing immediate benefits with long-term strategic partnerships. Additionally, dynamic game theory can help economists and policy-makers predict outcomes in environmental policy, where countries decide independently on investments in sustainable technologies.Moreover, in scenarios like auctions, such as the FCC spectrum auction, bidders use dynamic strategies to adjust their bids based on observed behavior. Understanding these complexities requires grasping the temporal component that dynamic games provide, offering a more nuanced view of competitive strategy in non-cooperative settings.
Real-world applications of non cooperative dynamic games often involve predicting competitor behavior and devising strategies that account for long-term implications, not just immediate outcomes.
Dynamic Game Theory Examples
Dynamic game theory provides insights into situations where players make sequential decisions. This sequential decision-making nature distinguishes dynamic games from static counterparts, making them highly applicable in real-life economics and strategic planning. Let's explore some classic and modern examples that show how dynamic games are used in theoretical and practical contexts.
Classic Examples of Dynamic Games
Classic dynamic games have helped shape our understanding of strategic interactions over time. Here are a few notable examples that illustrate the core principles:
- Cournot Competition: This model involves firms deciding quantities to produce over multiple periods. Each firm considers the output of the other before deciding its production level, aiming for a Nash equilibrium in quantities produced.
- Stackelberg Model: Here, the leader moves first and the follower observes before making a decision. This sequence allows the leader to anticipate the follower's response, leading to a strategic advantage and different outcomes compared to simultaneous moves.
- Repeated Prisoner's Dilemma: Unlike the one-shot version, players here interact over multiple periods. Cooperation might emerge as a rational response due to the ongoing nature of the game, with tit-for-tat strategies being frequently analyzed.
Imagine two firms, A and B, participating in a repeated pricing game:
- Stage 1: Firm A sets its price, \(P_A\).
- Stage 2: Firm B sets its price, \(P_B\), after observing \(P_A\).
Backward induction is a key tool in solving classic dynamic games, as it allows players to plan their strategies by starting from the end.
Modern Applications of Dynamic Game Theory
Modern applications of dynamic game theory reflect its flexibility and relevance in understanding strategic complexities in contemporary economic environments. With advancements in technology and global interconnectivity, dynamic games are applied in diverse fields such as:
- Environmental Policy: Countries make sequential decisions regarding greenhouse gas emissions, balancing current economic benefits against long-term environmental impact.
- Cybersecurity: Organizations and hackers engage in cat-and-mouse strategies, where defense mechanisms evolve based on observed breaches and anticipated attack techniques.
- Supply Chain Management: Suppliers adjust inventory levels dynamically based on changing consumer demand and competitor actions, aiming for just-in-time delivery.
One intriguing modern application is in the area of auction design, particularly with iterative auctions like the Combinatorial Clock Auction (CCA), used to allocate spectrum licenses. Participants bid over several rounds, adjusting their strategies based on previous results. This dynamic auction mechanism allows bidders to refine their valuations and strategies, ultimately leading to efficient allocation of resources. The structure of CCAs considers multiple interactions over time, embodying the essence of dynamic game theory by enabling strategic adaptations and responses. The analysis of such auctions involves evaluating bidder behavior, outcomes, and efficiency, providing insights into how dynamic frameworks can enhance complex economic systems.
Analysis of Equilibria in Dynamic Games
Understanding equilibria in dynamic games is crucial for analyzing how players make strategic decisions over multiple periods. These scenarios are multifaceted as players must anticipate future moves, responses, and the information available at each stage.
Equilibrium Concepts in Dynamic Games
Dynamic games can be analyzed through various equilibrium concepts, each tailored to capture the nuances of sequential decision-making.Subgame Perfect Equilibrium (SPE) is a refinement of Nash Equilibrium specific to dynamic games. It requires players to develop strategies that are optimal in every subgame, preventing any incentive to deviate at any stage.To find an SPE, you'll often use backward induction, starting from the last stage of the game and working backwards to determine the best actions for each previous stage.
Consider a two-stage game where two firms compete by setting quantities sequentially:
Stage | Firm A's Choice | Firm B's Response |
---|---|---|
1 | \text{Select } q_A | Observes |
2 | Observes | \text{Select } q_B |
The key to understanding equilibrium in dynamic games is examining how initial moves shape future actions and outcomes.
Importance of Dynamics in Potential Games
Dynamics introduce a critical layer of complexity in potential games, where each player's payoff changes due to others' strategies. In these games, strategic interactions are specifically framed within a potential function that aligns incentives and decisions.Potential games are characterized by the existence of a function where the incentive for all players to change their strategy can be encapsulated into a single metric, promoting the analysis of stability and equilibrium outcomes.
- Static Analysis: Each player's best response aligns with a potential function, simplifying equilibrium identification.
- Dynamic Considerations: Introduce adaptive behaviors, like learning dynamics, facilitating transitions toward equilibria over time.
Dynamic processes in potential games can reflect real-world phenomena like technology adoption or market entry. Take a market of competing technologies where firms continuously innovate. The introduction of new features triggers a dynamic process as competitors adjust to maintain market share. This scenario could be modeled through a potential game where innovation leads to shifts in equilibria, with firms dynamically adopting strategies that optimize their positions.In this adaptation context, you can model innovation incentives within a landscape shaped by a potential function reflecting aggregated market returns. As firms adjust strategy based on anticipated reactions, the market evolves toward points of dynamic stability, providing insights into strategic development and competitive innovation.
dynamic games - Key takeaways
- Dynamic Games Definition: Dynamic games in microeconomics involve strategic interactions over multiple periods where players consider past actions in decision-making.
- Characteristics of Dynamic Games: Key characteristics include unfolding in stages, strategy revision, backward induction, and categorization by information completeness.
- Non Cooperative Dynamic Game Theory: Focuses on independent, sequenced decisions by players aiming to maximize payoff without binding agreements.
- Analysis of Equilibria: Techniques like backward induction are used to find equilibriums like Subgame Perfect Equilibrium (SPE) in dynamic games.
- Examples and Real-World Applications: Classic examples include Cournot and Stackelberg models, while modern applications cover environmental policy, cybersecurity, and supply chain management.
- Dynamics in Potential Games: Dynamics, such as learning processes, guide players toward equilibria using a potential function to align incentives and decisions.
Learn faster with the 12 flashcards about dynamic games
Sign up for free to gain access to all our flashcards.
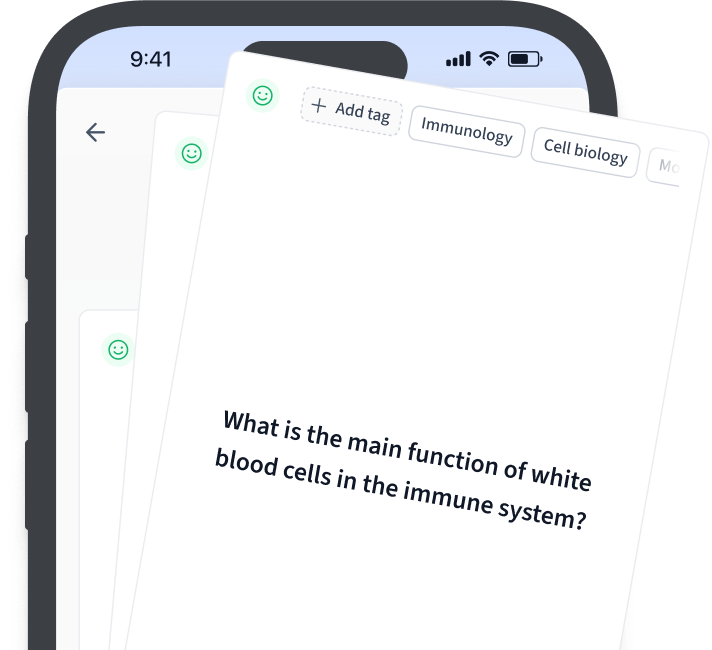
Frequently Asked Questions about dynamic games
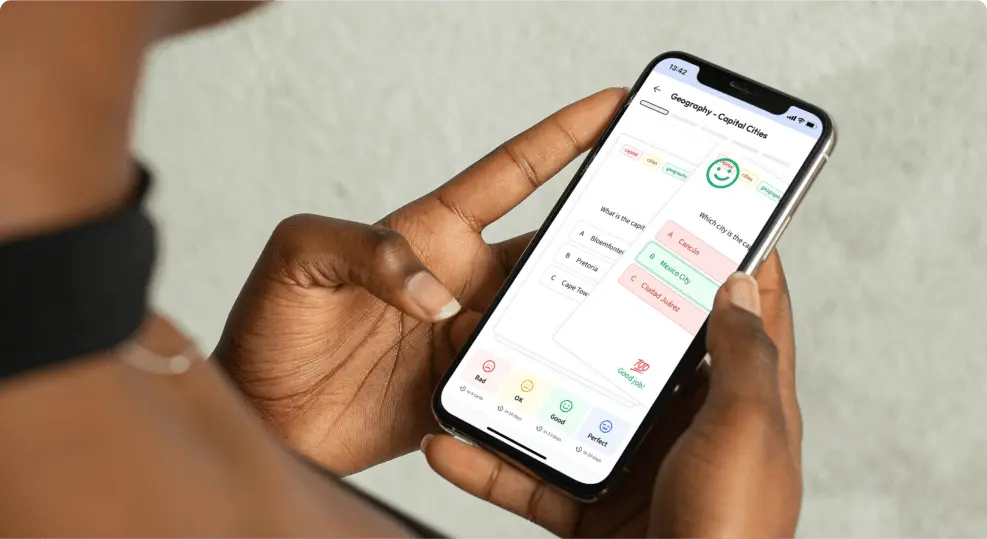
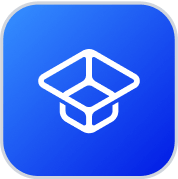
About StudySmarter
StudySmarter is a globally recognized educational technology company, offering a holistic learning platform designed for students of all ages and educational levels. Our platform provides learning support for a wide range of subjects, including STEM, Social Sciences, and Languages and also helps students to successfully master various tests and exams worldwide, such as GCSE, A Level, SAT, ACT, Abitur, and more. We offer an extensive library of learning materials, including interactive flashcards, comprehensive textbook solutions, and detailed explanations. The cutting-edge technology and tools we provide help students create their own learning materials. StudySmarter’s content is not only expert-verified but also regularly updated to ensure accuracy and relevance.
Learn more