Jump to a key chapter
Responsibility in AI Engineering
Artificial Intelligence (AI) is transforming industries and societies around the world. Understanding the concept of responsibility in AI engineering is crucial as AI plays a larger role in decision-making processes.
Responsibility in AI Engineering Definition
Responsibility in AI refers to the obligation of AI developers, engineers, and stakeholders to create, manage, and utilize AI systems in a manner that is ethical, trustworthy, and beneficial to society.
- Transparency: AI systems should be transparent in their operations and decision-making processes.
- Accountability: Ensuring that there are mechanisms in place to hold entities accountable for their AI systems' actions.
- Privacy: Respecting and protecting user privacy and data.
- Fairness: AI models should be free from bias and discrimination.
Example: An AI system used for hiring should evaluate candidates based on merit without bias towards gender, race, or ethnicity. Responsibility in AI engineering means designing the system to avoid any unfair treatment.
Accountability in AI Engineering Projects
Accountability is a cornerstone of responsibility in AI projects. It involves ensuring that AI systems and their developers can be held to account for the outcomes of AI application.
Key Aspects of Accountability:
- Defining clear roles and responsibilities for all stakeholders involved.
- Implementing regular audits and assessments of AI systems.
- Maintaining documentation of AI development processes.
The challenge of accountability in AI engineering projects lies in the complex and sometimes unpredictable nature of AI systems. For instance, when an AI algorithm makes a mistake, it is often difficult to trace back to any specific fault or individual. In such scenarios, distributed accountability frameworks may be required. This involves shared responsibility across developers, users, and organizations. By establishing clear accountability protocols, AI projects can ensure that stakeholders are aware of and committed to managing risks.
Responsibility of Developers Using Generative AI in Ensuring Ethical Practices
Generative AI, which can create content such as text, images, and music, presents unique ethical challenges. Developers must ensure their generative AI applications do not produce harmful or inappropriate content.
Here are some methods developers can use to ensure ethical practices:
Guideline | Description |
Limit usage | Implementing barriers to prevent misuse of generative AI in creating misleading or harmful content. |
Approval systems | Using human-in-the-loop systems to review and approve generated content before release. |
Error correction | Continuously training models to improve accuracy and reduce biases. |
When using generative AI, be cautious of creating content that could contribute to misinformation.
What is a Responsibility Gap in the Context of AI
The concept of a responsibility gap arises when it becomes unclear who is accountable for the actions or decisions made by AI systems. This ambiguity poses significant challenges in ensuring trustworthy AI application.
Understanding the Responsibility Gap
Responsibility gaps can occur when AI systems operate autonomously and make decisions without direct human intervention. These gaps are important to address because they can lead to accountability issues in the event of malpractice or errors.
Example: If an autonomous vehicle controlled by AI gets involved in an accident, determining who is responsible—the manufacturer, the software developer, or the owner—can be complex and unclear.
Responsibility gaps are more pronounced in systems where AI models independently adapt over time, such as with machine learning.
Deep Dive: In legal contexts, a responsibility gap can lead to unique challenges. Traditional liability laws are often inadequate for dealing with AI because they usually require pinpointing fault to a human actor. Some experts advocate for new legislation that captures the nuances of AI decision-making processes. However, devising such laws is complex, as it requires understanding all technical, ethical, and practical dimensions of AI deployment.
Addressing Responsibility Gaps in AI Systems
To bridge the responsibility gap, it is essential to establish clear accountability frameworks that detail the roles and liabilities of those involved in the AI lifecycle.
Action | Description |
Transparent systems | Designing AI systems whose actions can be easily understood and tracked by humans. |
Role assignment | Clearly defining the responsibilities of manufacturers, developers, and users in the AI process. |
Regulatory policies | Developing regulations that address AI's unique aspects and outline legal responsibilities. |
By tackling responsibility gaps proactively, industries can enhance trust in AI technologies and ensure they are used in ways that benefit society without causing unforeseen harm.
Ethical Implications of Autonomous Systems in Engineering
Autonomous systems are increasingly integral in various engineering domains, offering enhanced capabilities and efficiencies. However, their deployment raises important ethical questions that must be addressed to ensure their safe and fair operation.
Challenges in Autonomous Systems
The development and implementation of autonomous systems come with several challenges, especially regarding ethical considerations. Engineers and developers face complex decisions where the outcomes can have far-reaching consequences.
Autonomous Systems: These are systems that can perform tasks without human intervention, often relying on advanced algorithms and machine learning to adapt to their environments.
Challenges in autonomous systems include:
- Safety: Ensuring that autonomous systems can handle unexpected scenarios to prevent harm.
- Privacy: Balancing the need for data collection with the protection of individual privacy rights.
- Bias and Fairness: Addressing inherent biases in data and algorithms.
Deep Dive: One key challenge in autonomous systems is handling ethical dilemmas. Consider a self-driving car faced with an unavoidable collision scenario. The system must choose between minimizing harm to pedestrians or passengers. Such ethical dilemmas highlight the need for advanced decision-making frameworks that include ethical considerations during the design phase of autonomous systems. This requires collaboration between ethicists, engineers, policymakers, and the public to ensure well-rounded decision models.
When designing autonomous systems, prioritize safety and reliability above efficiency to prevent unintended consequences.
Ethical Decision-Making in AI
Ethical decision-making is a critical aspect when utilizing AI in engineering systems. AI systems must operate based on ethical principles and make decisions that align with societal values.
Principle | Description |
Transparency | AI systems should be open about how decisions are made, enabling users to understand the rationale behind them. |
Accountability | Mechanisms must be in place to hold developers and users accountable for AI outcomes. |
Fairness | AI should operate without bias, ensuring decisions do not unfairly disadvantage individuals or groups. |
Implementing ethical decision-making in AI involves:
- Building diverse datasets to train AI models.
- Conducting regular assessments to identify and correct biases.
- Establishing clear guidelines for the ethical use of AI.
Example: An AI-driven recruitment platform must ensure its algorithms do not favor or discriminate against candidates based on irrelevant factors like ethnicity or gender. Ethical decision-making can be achieved by refining the algorithm to focus solely on qualifications and experience.
Responsible AI Guidelines in Practice
Developing and implementing responsible AI guidelines is essential to ensure AI technologies are ethical, fair, and trustworthy. These guidelines provide a framework to guide AI developers, engineers, and companies as they design and deploy AI systems.
Implementing Responsible AI Guidelines
The implementation of responsible AI guidelines can be categorized into several key areas. Understanding and addressing these areas helps ensure AI systems are developed and utilized ethically.
- Transparency: AI systems should be designed with transparency in mind, allowing users to understand how decisions are made.
- Accountability: Develop mechanisms to ensure that responsible parties can be held accountable for AI actions and decisions.
- Fairness: Regularly evaluate AI models to check for biases and ensure they operate fairly.
- Privacy: Implement robust privacy measures to protect user data and comply with relevant regulations.
Example: A healthcare AI system should provide clear documentation on how it arrives at diagnoses. This transparency helps build trust among medical professionals and patients, ensuring the AI's recommendations are followed with confidence.
Deep Dive: One of the advanced techniques to implement responsible AI is through explainable AI (XAI). This approach focuses on creating AI systems whose decisions can be easily interpreted by humans. For instance, rather than simply outputting a decision, XAI tools can provide reasoning and context for each decision made by the AI. This capability is crucial in situations that require human oversight, such as financing and healthcare, where understanding the underlying reasoning of AI recommendations can prevent errors and increase acceptance.
Incorporate a feedback loop with stakeholders to continuously improve AI guidelines and address emerging ethical challenges.
Case Studies on Responsible AI Practices
Examining real-world case studies can provide valuable insights into how responsible AI practices can be implemented effectively and the impacts they have on various industries.
Here are some case studies demonstrating responsible AI practices:
Industry | AI Application | Responsible Practice |
Finance | Loan Approval | Ensuring algorithms do not discriminate based on race or gender. |
Healthcare | Diagnostic Tools | Employing XAI to make diagnostic processes transparent for clinicians. |
Retail | Customer Service | Implementing privacy measures to protect customer data during AI interactions. |
Example: In the finance sector, a bank implementing AI for loan approvals developed a system that consciously addressed bias by removing sensitive attributes from data inputs. This approach ensured that all applicants were treated equally based on relevant financial criteria.
Deep Dive: The healthcare industry often provides compelling examples of responsible AI practices. A notable case involves a hospital using AI-enabled diagnostic tools to assist radiologists. By implementing explainable AI, the hospital ensured doctors understood AI's conclusions, fostering collaborative decision-making. Moreover, feedback from these professionals was incorporated to continuously enhance the algorithm's accuracy. This deepened trust in AI technology and improved patient outcomes by combining AI and human expertise.
responsibility in ai - Key takeaways
- Responsibility in AI Engineering Definition: The obligation of AI developers and stakeholders to create and manage AI systems that are ethical and beneficial to society.
- What is a Responsibility Gap in the Context of AI: A situation where it is unclear who is accountable for AI system decisions or actions, leading to challenges in accountability.
- Accountability in AI Engineering Projects: Ensuring mechanisms are in place to hold parties accountable for AI system outcomes and operations.
- Responsibility of Developers Using Generative AI: Developers must ensure generative AI applications do not produce harmful content by implementing barriers and human review processes.
- Ethical Implications of Autonomous Systems: These include ensuring safety, privacy, and fairness, while addressing ethical dilemmas faced by systems like self-driving cars.
- Responsible AI Guidelines in Practice: Emphasize transparency, accountability, fairness, and privacy to ensure AI technologies are ethical and trustworthy.
Learn faster with the 12 flashcards about responsibility in ai
Sign up for free to gain access to all our flashcards.
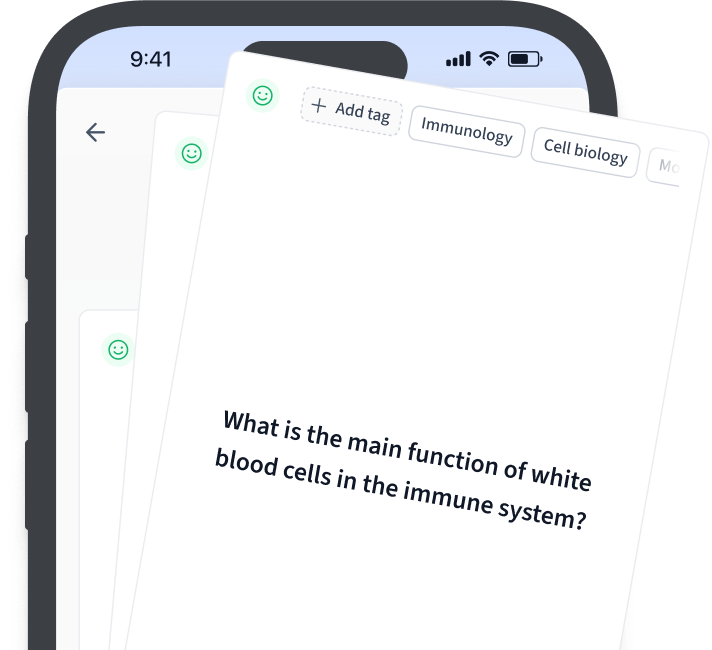
Frequently Asked Questions about responsibility in ai
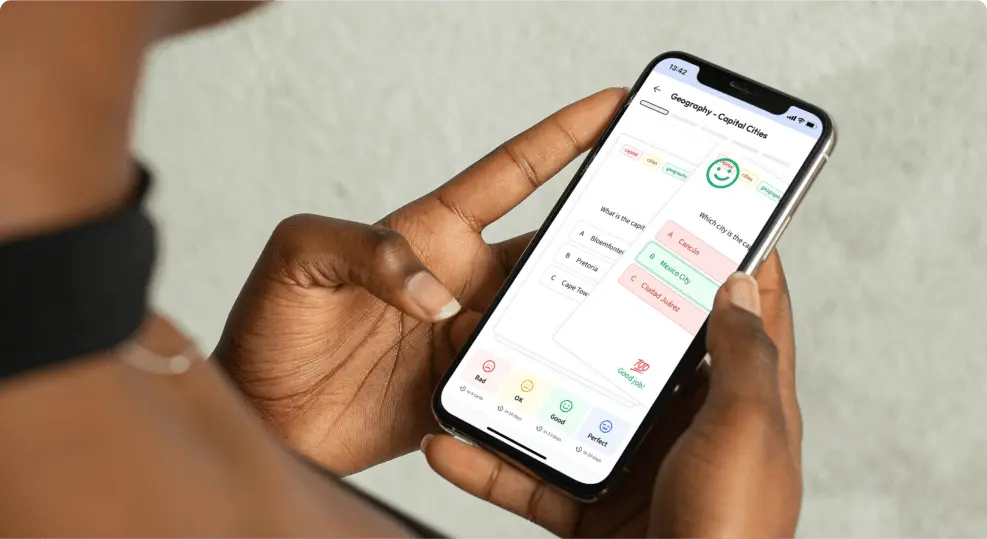
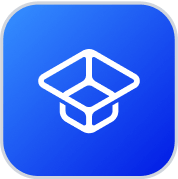
About StudySmarter
StudySmarter is a globally recognized educational technology company, offering a holistic learning platform designed for students of all ages and educational levels. Our platform provides learning support for a wide range of subjects, including STEM, Social Sciences, and Languages and also helps students to successfully master various tests and exams worldwide, such as GCSE, A Level, SAT, ACT, Abitur, and more. We offer an extensive library of learning materials, including interactive flashcards, comprehensive textbook solutions, and detailed explanations. The cutting-edge technology and tools we provide help students create their own learning materials. StudySmarter’s content is not only expert-verified but also regularly updated to ensure accuracy and relevance.
Learn more